Table of Contents
Healthcare is one of the most critical industries globally, and the use of machine learning in healthcare is revolutionizing the way healthcare is being delivered. Machine learning is a branch of artificial intelligence that has proven to be beneficial in healthcare analytics projects. In this article, we have discussed 8 real-time machine learning for healthcare analytics projects.
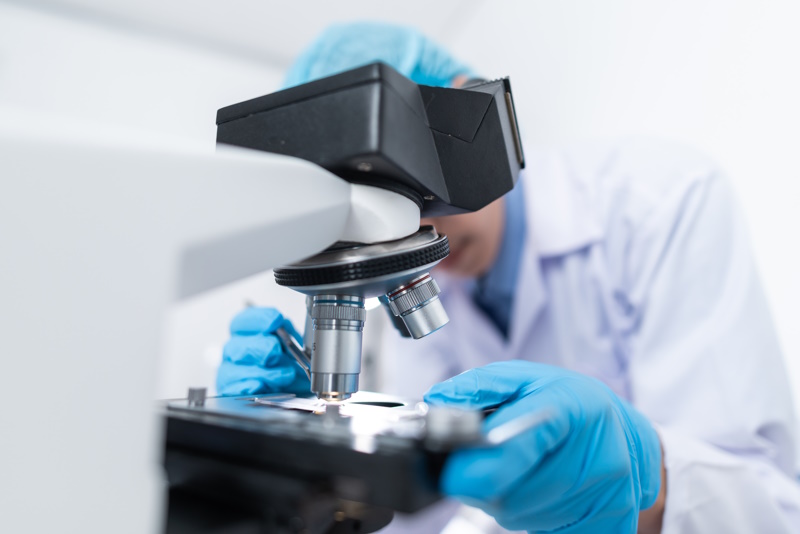
Healthcare providers have started leveraging machine learning to improve patient outcomes, disease diagnosis, analysis of Electronic Health Records (EHRs), reduce medical expenses, enhance operational efficiency, etc.
Machine Learning in Healthcare Statistics
The significance of machine learning in healthcare can be demonstrated through various statistics:
- According to a report by Grand View Research, the global machine learning market in healthcare is expected to reach $31.65 billion by 2025, with a CAGR of 44.5% from 2019 to 2025.
- A study published in the Journal of Medical Internet Research found that machine learning algorithms could improve the accuracy of predicting chronic diseases such as diabetes, hypertension, and coronary artery disease, with an accuracy rate of 84.7%.
- According to a report by Frost & Sullivan, the use of machine learning in drug discovery and development can reduce drug development costs by up to 60% and cut development time by up to 50%.
- A study published in the Journal of Digital Imaging found that machine learning algorithms could improve the accuracy of detecting breast cancer from mammography images by up to 9.4%.
- A report by the Association of Fraud Examiners found that healthcare fraud costs the industry approximately $68 billion annually. Machine learning algorithms can help healthcare providers detect and prevent fraudulent health insurance claims, resulting in significant cost savings.
These statistics demonstrate the significant impact that machine learning is having on the healthcare industry.
Machine Learning for Healthcare Analytics projects
1. Prediction of Breast Cancer
The use of machine learning has the capability to predict breast cancer by identifying hidden features within the data. As we know, machine learning usually works on numerical data, so in this use case, numerical features can be calculated from a digitized image of a fine needle aspirate (FNA) of a breast mass, and then machine learning algorithms can be applied for predicting whether the breast cancer is malignant and benign. This is the most significant machine learning for healthcare project.

Incorporating several risk factors into a breast cancer prediction model can aid in the early detection of the disease and facilitate the development of appropriate care plans.
Supporting Article: Breast Cancer prediction using Machine Learning
Supporting Dataset: Breast Cancer Wisconsin dataset
2. Prediction of Chronic Kidney Disease
Millions worldwide are impacted by chronic kidney disease (CKD), a crucial health concern that can result in severe complications. Timely identification and treatment can considerably enhance patient outcomes. In recent years, machine learning has emerged as an effective means of predicting CKD.
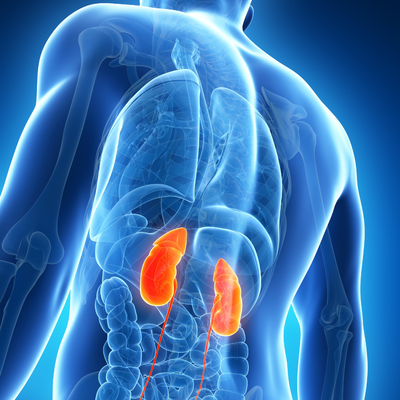
In this use case, machine learning can be applied based on different health indicators and demographic information of the patients for predicting early-stage chronic kidney disease.
Supporting Article: Chronic Kidney Disease Detection Using Logistic Regression
Supporting Dataset: Chronic Kidney Disease dataset
3. Early-Stage Diabetes Detection using Machine Learning
Diabetes mellitus is a metabolic disorder characterized by elevated blood sugar (glucose) levels in the body. The International Diabetes Federation predicts that the number of individuals with diabetes will increase to 643 million by 2030 and 783 million by 2045.

Machine learning can be applied effectively for detecting early-stage diabetes using certain medical predictor variables. The benefits of applying machine learning for diabetes detection include early detection, improved accuracy, personalized treatment, cost savings, and enhanced efficiency.
Supporting Article: Prediction of Diabetes Using Machine Learning
Supporting Dataset: Diabetes Dataset
4. Heart Disease Detection
Heart disease occurs due to the blockage of arteries that supply blood to the heart with plaque, made up of cholesterol and other substances that narrow and harden the arteries, leading to reduced blood and oxygen supply to the heart, ultimately causing heart attack or stroke.

Machine learning has shown significant results in predicting heart disease using medical as well as demographic features of the patient. The benefits of applying machine learning in heart disease include early detection, personalized treatment, and improved accuracy in predicting the risk of heart disease.
Supporting Article: Heart Disease Detection Using Machine Learning
Supporting Dataset: Heart Disease Dataset (Comprehension)
5. Medical Insurance Premium Prediction
Machine learning can be applied to predict medical insurance premiums by analyzing data from medical histories and lifestyle factors, resulting in more accurate predictions and better risk management. This can help insurance providers develop personalized insurance plans and reduce costs for both providers and customers.
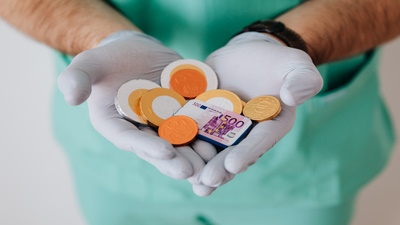
Supporting Article: Predicting Medical Insurance Premiums Using Machine Learning
Supporting Dataset: Insurance Premium Prediction Dataset
6. Lung Cancer Detection using Machine Learning
Machine learning can be applied to predict the likelihood of lung cancer in individuals based on their medical history, lifestyle factors, and genetic predisposition. By leveraging machine learning for lung cancer detection, healthcare providers can improve patient outcomes, reduce healthcare costs, and facilitate the development of more effective treatment plans.
Supporting Dataset: Thoracic Surgery Dataset
7. Liver Disease Detection Using Machine Learning
Machine learning can be applied to detect liver disease, which can aid in early diagnosis and management. Machine learning algorithms can analyze patient data, including medical history, laboratory results, and imaging data, to identify patterns and predict the likelihood of liver disease. This can enable healthcare providers to develop personalized treatment plans for patients, resulting in better patient outcomes and reduced healthcare costs.
Supporting Dataset: ILPD Dataset
Supporting Article: Liver Disease Prediction (Kaggle)
8. Drug Recommendation System
A drug recommendation system that utilizes a dataset of user reviews regarding drugs can be developed to classify medical conditions and recommend the top three drugs based on average user ratings and the total number of ratings. By analyzing the data, machine learning algorithms can identify patterns in drug efficacy for various medical conditions, allowing for more accurate drug recommendations.

Additionally, the system can help healthcare providers personalize treatment plans for patients based on their medical condition, improving patient outcomes and satisfaction. By leveraging machine learning for drug recommendations, healthcare providers can enhance patient care and ensure that patients receive the most effective treatments for their medical conditions.
Supporting Article: Drug Recommendation System Using NLP & Machine Learning
Supporting Dataset: Drug Review Dataset
Conclusion
Machine learning has revolutionized the healthcare analytics domain, and its benefits are evident in the various use cases discussed in this article. From electronic health records management to disease diagnosis and prognosis, machine learning algorithms have proven to be a valuable tool for healthcare providers. By leveraging machine learning, healthcare providers can improve patient outcomes, reduce costs, and enhance operational efficiency. In this article, we have discussed 8 real-time projects in machine learning for healthcare domain along with the supporting article and dataset which will help to get started in the project and you can also use them as your portfolio projects for building your profile in machine learning.
Machine Learning For Healthcare Analytics Project – Tweet This